Pega Certified Decisioning Consultant (PCDC) Interview Questions
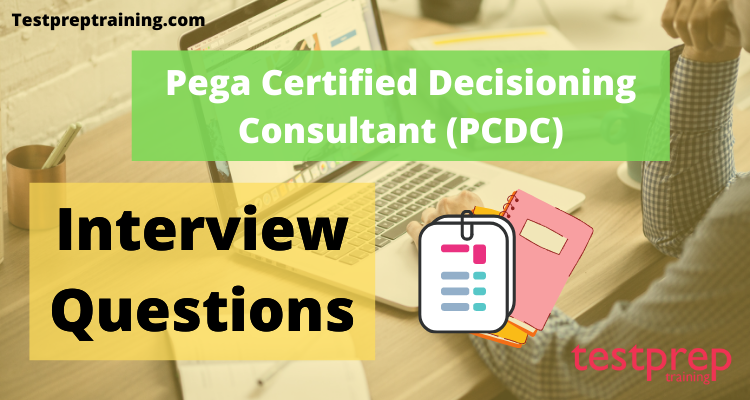
Interview preparation is just as crucial as exam preparation. As a result, preparing for an interview necessitates far more practice, time, effort, and confidence than preparing for any other exam. You only get one chance to make a good first impression, so do your best. As a result, we have done our best to provide you with the best and most expert-revised interview questions to help you prepare successfully for the Pega Certified Decisioning Consultant (PCDC) interview. In addition, we’ve covered all Pega Certified Decisioning Consultant (PCDC) Interview Questions, from beginner to advanced. So, we strongly advise applicants to prepare with the finest and accomplish the best.
Given Below are some top Pega Certified Decisioning Consultant (PCDC) Interview Questions. This would help the candidates get an idea about what types and patterns they should expect and prepare accordingly.
Advanced Interview Questions
What experience do you have with Pega Decisioning and how have you used it to help organizations make better decisions?
Pega Decisioning is a suite of applications built on the Pega Platform that enables organizations to make informed, data-driven decisions. As a Pega Certified Decisioning Consultant, I have extensive experience in designing, developing, and implementing Pega Decisioning solutions for various clients across multiple industries.
In my work, I have leveraged Pega Decisioning to help organizations automate their decision-making processes, enabling them to make better decisions in real-time. One example is a project I worked on for a financial services client where I used Pega Decisioning to automate their credit underwriting process. The solution integrated with the client’s existing systems to access relevant customer data and used Pega’s advanced analytics and machine learning algorithms to make risk assessments. This resulted in a significant reduction in the time required to make a credit decision and improved the accuracy and consistency of the underwriting process.
Another example is a project I worked on for a retail client where I used Pega Decisioning to automate their customer engagement process. The solution analyzed customer data to determine the best way to engage with each customer, whether through email, phone, or other channels. The solution also used advanced analytics to determine the best message to send and the best time to send it, resulting in a significant increase in customer engagement and customer satisfaction.
In conclusion, my experience with Pega Decisioning has allowed me to help organizations make better decisions by automating and optimizing decision-making processes using advanced analytics and machine learning algorithms. I have a strong understanding of the Pega platform and a proven track record of delivering successful Pega Decisioning projects for clients in a variety of industries.
Can you explain how Pega Decisioning integrates with other Pega tools and systems?
Pega Decisioning is a component of the Pega Platform and integrates with other Pega products and tools to deliver end-to-end decisioning capabilities.
- Pega Marketing: Pega Decisioning integrates with Pega Marketing to provide advanced targeting and personalization capabilities. With this integration, Pega Decisioning can leverage customer data from Pega Marketing to deliver relevant and personalized experiences to customers.
- Pega Customer Service: Pega Decisioning integrates with Pega Customer Service to provide real-time decisioning for customer interactions. This integration enables customer service representatives to make informed decisions based on customer data, past interactions, and current context.
- Pega Robotics: Pega Decisioning integrates with Pega Robotics to automate decision-making processes and improve operational efficiency. For example, Pega Decisioning can be integrated with Pega Robotics to automate the decision-making process for fraud detection in real-time.
- Pega Customer Decision Hub: Pega Decisioning integrates with Pega Customer Decision Hub to provide a centralized repository of decisioning rules and policies. This integration enables organizations to manage their decisioning rules and policies in a single location, making it easier to maintain consistency and transparency across the enterprise.
In summary, Pega Decisioning integrates with other Pega products and tools to provide a comprehensive decisioning solution that enables organizations to make informed decisions and deliver personalized experiences to their customers.
What are the key features and benefits of Pega Decisioning and how do they contribute to a successful decisioning strategy?
Pega Decisioning is a cloud-based decision management platform that leverages advanced artificial intelligence, machine learning, and predictive analytics to help organizations make smarter, faster decisions. It has been designed to help businesses streamline their decision-making processes, improve customer engagement, and drive growth. The key features and benefits of Pega Decisioning include:
- Predictive analytics: Pega Decisioning offers predictive analytics and machine learning algorithms to analyze data and make predictions about customer behavior and preferences. This helps organizations make informed decisions that are tailored to individual customers, resulting in more personal and relevant experiences.
- Customer insights: Pega Decisioning provides real-time customer insights that are based on both structured and unstructured data. This enables organizations to make data-driven decisions that are based on a complete view of the customer, including their behavior, preferences, and needs.
- Real-time decision making: Pega Decisioning enables organizations to make real-time decisions based on the latest customer data, reducing the time it takes to respond to customer needs. This leads to faster and more effective decision-making, which can result in increased customer satisfaction and loyalty.
- Improved customer engagement: Pega Decisioning helps organizations to create personalized and relevant experiences for their customers, improving engagement and driving growth. By using predictive analytics and machine learning algorithms, organizations can anticipate customer needs and provide relevant offers, recommendations, and messages in real-time.
- Integration with existing systems: Pega Decisioning integrates seamlessly with existing systems, allowing organizations to leverage existing data and processes to make better decisions. This helps to streamline decision-making and eliminate the need for manual processes, reducing the risk of errors and increasing efficiency.
In conclusion, Pega Decisioning is a powerful platform that offers a range of benefits for organizations looking to improve their decision-making processes. With its advanced predictive analytics, real-time decision making, and improved customer engagement capabilities, Pega Decisioning helps organizations to make data-driven decisions that are tailored to individual customers, leading to increased satisfaction, loyalty, and growth.
Can you discuss your experience with creating and deploying decisioning strategies in a Pega environment?
I have extensive experience in creating and deploying decisioning strategies in Pega environments. This includes designing and implementing rule-based decisioning strategies, such as scoring and targeting models, as well as predictive models. I would have a solid understanding of Pega’s decisioning framework and be proficient in using Pega’s decision management tools, such as the Decisioning composer and the Decision Tables.
In my role, I would work closely with business stakeholders to understand their decisioning requirements and design and implement solutions that meet those requirements. I would also be responsible for testing and fine-tuning decisioning models to ensure they are functioning as intended and delivering optimal results.
Additionally, I would have experience deploying decisioning models in a production environment, including implementing security and governance controls to ensure that the models are used appropriately.
Can you explain the difference between Pega Decisioning and other decision management tools?
I would say that Pega Decisioning is a comprehensive, end-to-end decision management platform that enables organizations to automate and optimize their business decisions. It provides a unified platform to model, execute and continuously improve business decisions, which helps organizations to provide consistent, relevant and personalized experiences to their customers. In comparison to other decision management tools, Pega Decisioning offers several unique features and benefits, including:
- Built-in Case Management – Pega Decisioning integrates with Pega’s Case Management framework, providing a unified platform for both process automation and decision management.
- AI-Driven Decisioning – Pega Decisioning leverages Pega’s AI capabilities, including Predictive and Adaptive models, to automate and optimize business decisions in real-time.
- Business User Empowerment – Pega Decisioning provides a business-friendly interface, allowing business users to manage and update decision rules without IT involvement.
- Continuous Improvement – Pega Decisioning provides the ability to monitor and optimize decision performance, enabling organizations to continuously improve their decision making over time.
In conclusion, Pega Decisioning provides a comprehensive and unified platform for automating and optimizing business decisions, with a focus on empowering business users and enabling continuous improvement.
Can you describe a project where you used Pega Decisioning to improve business outcomes and how did you measure the results?
Yes, I recently worked on a project for a large financial services company where we leveraged Pega Decisioning to improve business outcomes. The company was looking to enhance its customer engagement strategy and personalize its marketing efforts.
We used Pega Decisioning to build a customer-centric decisioning model that analyzed customer behavior and preferences. The model was integrated with the company’s customer data and marketing systems to create a unified customer view.
We then used the model to create targeted and personalized marketing campaigns that were delivered to customers through various channels, including email, SMS, and push notifications. To measure the results, we conducted a thorough analysis of customer engagement metrics, including open rates, click-through rates, and conversion rates. We also conducted customer surveys to gauge customer satisfaction and feedback. The results were outstanding, with a significant increase in customer engagement and conversion rates. The personalized marketing campaigns led to higher customer satisfaction and increased customer loyalty, which ultimately translated into increased revenue for the company.
In conclusion, Pega Decisioning played a crucial role in helping the financial services company improve its business outcomes by enabling personalized and targeted marketing efforts that resulted in increased customer engagement and revenue.
Can you explain how Pega Decisioning works with big data and predictive analytics to support decision making?
Pega Decisioning is a platform that leverages big data and predictive analytics to provide organizations with real-time, data-driven decision-making capabilities. It integrates with a variety of data sources, such as customer behavior, business processes, and internal and external data sources to provide a complete picture of the customer.
Pega Decisioning uses predictive analytics algorithms to analyze this data and identify patterns and trends that can help organizations make better decisions. For example, it can analyze customer behavior data to predict which customers are most likely to churn, which products are most likely to sell, and which marketing campaigns are most likely to be successful.
Once the data is analyzed, Pega Decisioning can use the insights generated to make decisions in real-time, based on pre-defined rules and decision logic. For example, it can determine the next best offer to make to a customer, or which channel to use to reach them.
In conclusion, Pega Decisioning leverages big data and predictive analytics to provide organizations with a comprehensive view of their customers, and the ability to make data-driven decisions in real-time, based on pre-defined rules and decision logic.
How do you ensure that Pega Decisioning models are accurate and updated regularly to reflect changes in the business environment?
As a Pega Certified Decisioning Consultant, ensuring the accuracy and regular updates of Pega Decisioning models is a critical aspect of delivering optimal business outcomes. Here are the steps I follow to achieve this:
- Monitoring the Business Environment: Regular monitoring of the business environment helps to identify any changes that may impact the decisioning models. This includes keeping track of changes in customer behavior, market trends, business processes, and regulatory requirements.
- Conducting User Acceptance Testing (UAT): Before deploying any decisioning model, I conduct a thorough UAT to ensure that the model works as expected and accurately reflects the current business environment. This includes testing the model with different scenarios and validating the results.
- Collaborating with Stakeholders: Collaborating with stakeholders, including business owners and subject matter experts, helps to ensure that the decisioning models accurately reflect the current business environment and meet the needs of the organization.
- Using Automated Tools: Automated tools, such as Pega Decisioning Analytics, can help to validate the accuracy and performance of decisioning models. These tools can be used to analyze the results of UAT, identify any inaccuracies or deviations, and make necessary updates.
- Regularly Reviewing Models: Regularly reviewing and updating decisioning models helps to ensure that they remain relevant and accurate. This includes updating decisioning models to reflect changes in the business environment, such as changes in customer behavior, market trends, and regulatory requirements.
By following these steps, I can ensure that Pega Decisioning models remain accurate and updated regularly, which helps to deliver optimal business outcomes.
Can you discuss your experience with creating and maintaining Pega Decisioning rule sets and how you ensure compliance with regulations and policies?
As a Pega Certified Decisioning Consultant, I have extensive experience in creating and maintaining Pega Decisioning rule sets. This includes developing, testing, and implementing decisioning rules for various use cases and business processes. I have worked with multiple clients to design and implement decisioning rules that drive automated decisions and recommendations based on customer interactions, transactions, and behavioral data.
In terms of ensuring compliance with regulations and policies, I have implemented various processes and controls to ensure that the decisioning rules adhere to all relevant laws, regulations, and organizational policies. This includes performing regular audits of the rule sets, conducting impact assessments, and implementing monitoring and reporting mechanisms to detect and address any potential violations.
Additionally, I work closely with clients to understand the specific regulatory and policy requirements, and integrate these requirements into the decisioning rules to ensure that they are followed consistently and accurately. I also stay up-to-date with the latest developments in regulations and policies to ensure that my clients’ systems remain compliant.
Overall, I have a strong commitment to compliance and am experienced in designing and implementing decisioning rule sets that are both effective and compliant with all relevant regulations and policies.
Basic Interview Questions
1. What is a Proposition?
A proposition can be anything you provide to your customers, such as goods, services, or advertising. Propositions in the Pega Platform have the option to arrange under a three-level hierarchy: business issue, group, and proposition. Each proposition comes with a unique identifier by combining these levels. This corporate hierarchy can be customized to represent your current products and services.
2. How can you ensure that your simulations are accurate?
You can test a sample of your production data in a dedicated simulation environment to guarantee that your simulations are accurate enough to assist you to make key business choices. You can forecast the impact of modifications to your decision logic by running simulations on sample production data before implementing them in your live production environment.
3. What are Data Flows?
Data flows are a scalable, adaptable approach for simultaneously managing various decisions that follow a simple input-process-output pattern.
4. When do you use Event Strategies?
You can utilize event methods to identify the most essential opportunities and dangers in choosing the next best actions for your customers by detecting interactions such as Call Detail Records, prepaid balance recharges, or credit card transactions.
5. What is Revision Management?
You utilize revision management to adapt swiftly to internal issues as well as changes in the external environment that have an impact on your business. For example, a corporation can respond more quickly to changes in client behavior by updating the choice strategies and propositions that define its next-best-action decision framework. Furthermore, they let business users make and test changes to their applications outside of traditional enterprise release cycles.
6. what happens when you use Data Flow to call a Strategy?
You can separate the business process from the decision strategies by utilising a data flow to call them. This eliminates the requirement to update one component when the other changes.
7. What is the purpose of Real-Time Data Grid Service?
For your next-best-action strategies, the Real-Time Data Grid (RTDG) service does historical analysis and predictions. You can track the outcomes of your next-best-action decisions in real time with the RTDG service.
8. Define Stream Service.
The Stream service enables the asynchronous flow of data between processes in the Pega Platform. The Stream service is a multimode component that is based on Apache Kafka.
9. How do you start a decision management service?
To start a decision management service, you provide a node type appropriate to that service to an existing node; for example, you assign the DDS node type to a selected node to start the Decision Data Store (DDS) service. Any Pega Platform node can have a decision management service node type assigned to it. You can scale a decision management service horizontally by assigning the corresponding node type to extra nodes after it has begun. The number of nodes for each service is determined by the scalability and resiliency needs of your application.
10. How do you ensure scalability in decision management service?
To ensure the scalability of each service, you assign only one node type to a node.
11. Why do you build extraction models?
You build an extraction model to identify a word or a phrase as structured content and associate metadata with that content.
12. Define Naïve Bayes Algorithm.
Naive Bayes is a simple but effective algorithm for predictive modeling, with strong independence assumptions between the features. It is the fastest modeling technique.
13. What is a Support Vector Machine?
SVM is a discriminative classifier. It handles nonlinear data by using kernel mapping, implicitly mapping kernel inputs to high-dimensional feature spaces. SVM models are the slowest to build.
14. How is Precision, Recall and F-score calculated?
After creating a model, look at accuracy, recall, and F-score measures, which are calculated by comparing the labels you predicted (the manual outcome) with the labels the model predicted (the machine outcome) for each test record. The following formulas are for calculating these accuracy measures:
- Precision – The number of true positives divided by the count of machine outcomes (labels that the model predicted).
- Recall – The number of true positives divided by the count of manual outcomes (labels that you predicted).
- F-score – The harmonic mean of precision and recall.
15. What is Decision Data Store (DDS) service?
The Decision Data Store (DDS) service manages the Cassandra cluster and stores decision management data in a Cassandra database.
16. When can you do Partitions without a leader?
When all brokers hosting replicas for this partition are offline, or no synchronised replica can take leadership owing to message count difficulties, partitions without a leader can occur. The Stream service does not process messages for a partition when it is down.
17. What are Under-replicated and Offline Partitions?
- Under-replicated: The number of partitions that do not synchronize with the leader node. For example, under-replication can occur when a Stream node fails.
- Offline: The number of partitions that do not have a leader.
18. Explain different types of Data Flows?
- Batch data flows: Go over a finite set of data and eventually complete processing. Batch data flows are mainly used for processing large volumes of data.
- Real-time data flows: Go over an infinite set of data. Real-time data flows are always active and continue to process incoming stream data and requests.
- Single case data flows: Executed on request with the data flow source set to abstract. Single case data flows are mostly to process inbound data.
19. What is a Prediction Studio?
Prediction Studio is an authoring environment that allows you to manage the entire life cycle of AI and machine-learning models, including model creation, monitoring, and updating. You can also handle other resources from Prediction Studio, such as data sets, taxonomies, and sentiment lexicons.
20. Define Kafka?
The Kafka data set is a high-throughput, low-latency platform for dealing with real-time data feeds that can be used as input for Pega Platform event strategies.
21. Explain Kafka Datasets?
In terms of event and message queueing, Kafka data sets are known for their great performance and horizontal scalability. Data sets in Kafka can be partitioned to distribute load throughout the cluster.
22. Explain Decision Strategy Manager?
Pega Customer Decision Hub is built on Decision Strategy Manager (also known as DSM), a Pega Platform capability that provides decision management technology such as proposal management, Interaction History, Adaptive Decision Manager, decision strategies, and other capabilities. Organizations who want to employ decisioning in customer service and process automation should use Decision Strategy Manager.
23. Why should you perform simulation Tests?
You can run strategies of different complexity on a pre-selected sample group of clients using simulation testing. You may simulate the outcome of your decision management framework by making millions of decisions at the same time. Further, you may visualize the results of a simulation test in Scenario Planner, where you can examine whether the new approach provides the intended result.
24. Define Page Header in Prediction Studio.
The top of the screen displays the name of the current work area, such as Predictions, and allows you to conduct a variety of typical tasks such as examining model reports, deleting models, and so on. You can also use this toolbar to add models or other resources.
25. What is the Event Strategy Rule?
Event strategies are a way to make complex event processing tasks easier. You define event patterns, search for them in a data stream, and react to the patterns that emerge. From real-time data through the final emit command, event strategies are sequenced using a collection of instructions and execution locations. You can apply filter, window, aggregate, and static data instructions to real-time data and emit.
26. What are the different types of declarative rules?
Declare Expressions, Declare Constraints, Declare On change, Declare trigger, Declare Index.
27. What is Summary View Rule?
A summary view rule defines a two-level report presentation, displaying summary counts, totals, or averages at first and allowing users to drill down to supporting detail for that row by clicking a row. Interactive charts, trend reports, and the usage of AJAX for pop-up Smart-Info windows are all supported by summary view rules.
28. What is the purpose of Paging in Listview?
To divide the ListView into different pages and set the number of records to be displayed on a page.
29. Give some benefits of Event Catalog.
You can use the Event Catalog to construct various events that collect data from certain data sources (Data set or Report definition) and store it in the Event Store data set. You may also use this information to collect information about client interactions and present it in an events feed that you can add to your user experience.
30. How do you enable languages for NLP?
- In the navigation pane of Prediction Studio, click Settings Prediction Studio settings .
- In the Languages section, click Edit languages.
- And, in the Language selector window, select the languages that you want to enable in your text models.
- Click Save.
31. When is Listview used?
List view is generally used for complex queries where sorting is required and also we can retrieve less information using Paging.
32. Why do you need to Filter Incoming Data?
Data is filtered to reduce the number of records that your data flow needs to process. Reducing the number of records that your data flow needs to process, decreases the processing time and hardware utilization.
33. How do you delete Unversioned Propositions?
- In the header of Dev Studio, click Configure Decisioning Decisions Proposition Management .
- On the Proposition Management landing page, click the Unversioned proposition data tab.
- Select a proposition or propositions that you want to delete and click Delete.
- In the Delete dialog, click Delete to confirm.
34. How do you delete Versioned Prepositions?
- In the header of Dev Studio, click Configure Decisioning Decisions Proposition Management Hierarchy .
- In the Decision data records list, click the group that contains the proposition that you want to delete.
- Select the proposition or propositions that you want to delete and click Delete.
35. When do you perform a Single Case Run?
You perform a single case run to test your strategy against a specific record. You can test whether the strategy that you created is set up correctly and delivers expected results.
36. What is an Agent?
An agent is a server’s internal background process that performs actions on a regular basis. The itineraries of the agents are determined by the regulations in our programme. Agents also conduct system activities including delivering e-mail notifications about assignments and outgoing correspondence, updating full-text search indexes, syncing caches across nodes in multi-node systems, and so on.
37. What types of validations are there?
- Client Side Validations
- Server Side Validations
38. Explain Decision statistics.
- The highest ranked decisions – indicates the most popular propositions.
- The lowest ranked decisions – indicates the least popular propositions.
- Number of records with decisions – indicates the number of records and per cent of the total number of records associated with at least one decision for each shape and in the strategy.
39. What is the Page copy method?
To copy the contents of a source clipboard page to a new or previously generated destination clipboard page, use the page-copy method. The source page has not been changed. The destination page contains properties copied from the source page, as well as extra properties from a model when this procedure completes.
40. Explain the RDB-Save method?
RDB-Save method is used to save the contents of a clipboard page into a row of a relational database. The system saves the properties on the specified step page to the specified table in the database. This method operates in conjunction with a Connect SQL rule that contains SQL statements such as INSERT, UPDATE, and CREATE statements on the Save tab.